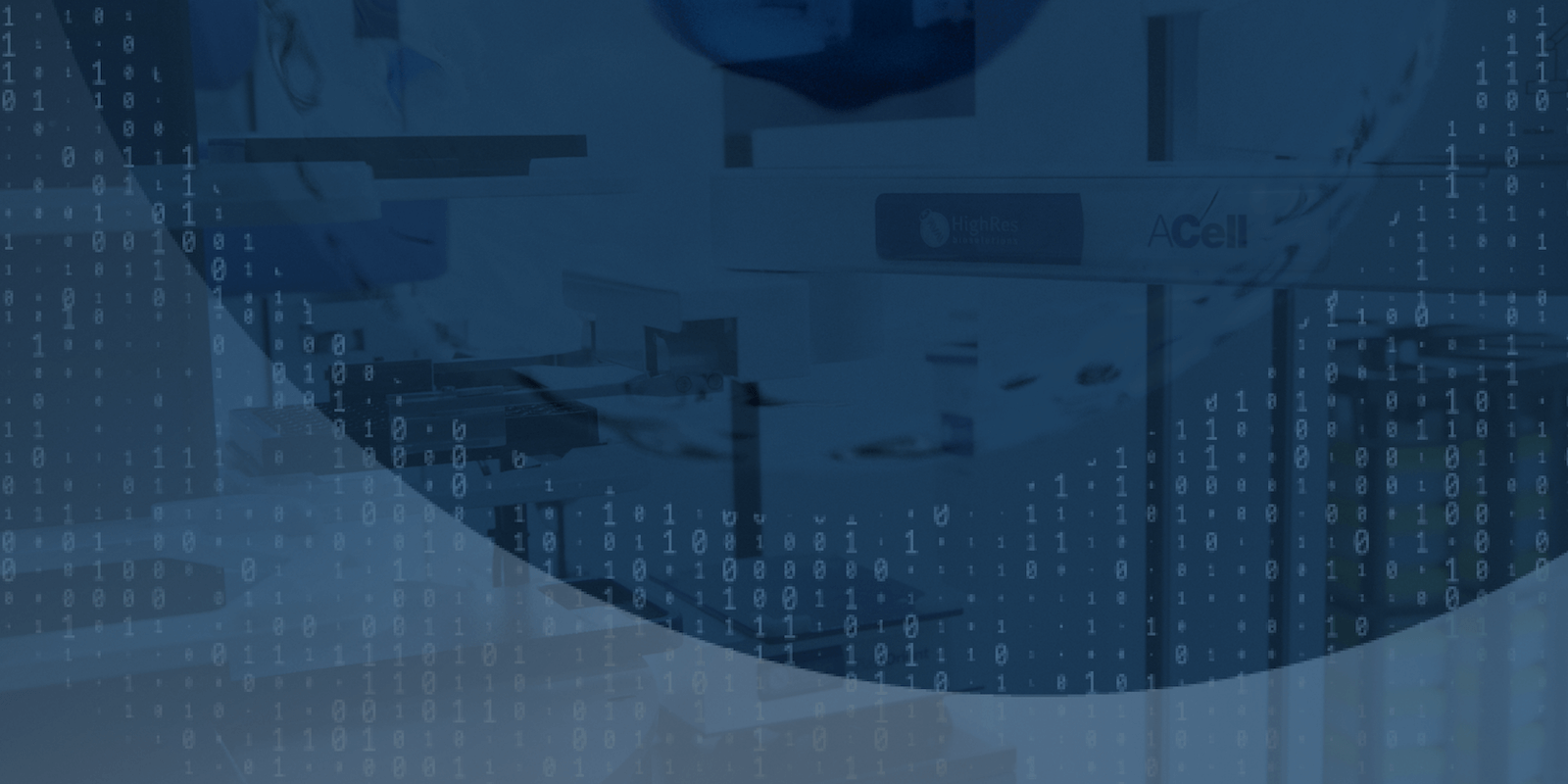
Predicting and Confirming Drug Synergies Against Cancer Cell Lines or Individual Tumor Samples Utilizing AI Software and HighRes Lab Automation
Learn how a deep learning predictive modeling approach, confirmed by high throughput screening, can identify drug synergies against tumors.
Anti-cancer therapies using multiple drugs are proven effective in improving cancer outcomes. At the same time, identifying a specific and impactful drug cocktail against a given cancer cell type and individual patients remains a challenge; even more so when treating heterogeneous tumors.
The pre-print article, PAIRWISE: Deep Learning-based Prediction of Effective Personalized Drug Combinations in Cancer, describes a deep learning (DL) computational modeling method, called PAIRWISE, to accurately predict synergistic drug combinations for use with cell lines and patient profiles.
What is Deep Learning?
In the artificial intelligence (AI) space, machine learning (ML) models can learn functional maps between input data and drug synergies only after data is pre-processed and the model undergoes human-directed training. As a subset of ML, DL uses neural networks to autonomously learn from large data sets. Without human involvement, DL uses learnings to make decisions and predict outcomes. A good degree of accuracy can be challenging when using DL with small data sets, like cancers with limited cases.
What is PAIRWISE?
PAIRWISE is a DL model developed by researchers from Weill Cornell Medicine and AstraZeneca to consider multiple modalities that can influence cancer drug responses. These feature sets include chemical structure, drug target, and individual tumor sample transcriptomes. PAIRWISE adds transfer learning into the assessment. This means that it is pre-trained on large drug and transcriptome datasets so that it can learn general representations of both compound chemical structures and sample-specific transcriptomes.
In essence, this means that PAIRWISE may be a significant tool to accurately predict personalized synergistic drug combinations that can be added to individual patient cancer treatment strategies
Testing Drug Prediction Performance
The authors applied PAIRWISE to publicly sourced screening data including 279,452 unique drug combinations, 2614 drug compounds, and 167 cancer cell lines taken from diverse tissue samples. Compared to results from other published DL models, PAIRWISE demonstrated superior predictive performance.
Highres HTS System Confirms PAIRWISE Prediction Robustness
The team also used PAIRWISE to predict drug combination synergies in primary patient lymphoma samples. This DL model predicted 30 out of 268 drug combination synergies.
Out of those 30 predicted drug combinations, 21 were selected to screen against established lymphoma cell lines using a fully integrated and automated work cell from HighRes Biosolutions. Before they were screened in combination, each drug’s activity was assessed to characterize synergistic effects on specific cell lines.
Work cell hardware included a Prime® automated liquid handler to seed lymphoma cells into 384-well microplates and another liquid handler to manage compound starting concentrations and serial dilutions. The automated system also included a MicroSpin™ automated centrifuge, microplate incubators, and a multi-mode plate reader.
Data from the high throughput screens confirmed that the PAIRWISE synergistic drug combinations were “strongly and significantly enriched for synergistic cell killing outcomes…” Additionally, a high concordance was seen between median predicted drug synergies in lymphoma patients and median experimental synergy scores in lymphoma cell lines. Taken together, this confirms the robustness of the PAIRWISE predictions.
Robust Lab Operations Empowered Through Cellario
PAIRWISE wasn’t the only robust part of this study. CellarioScheduler Lab Automation Software was used to streamline the high throughput screening workflow with robust control of all devices in the work cell along with hands-free efficiency.
With Cellario, users can design, edit, and simulate experimental protocols, like the CellTiter-Glo® (Promega) methods used in this PAIRWISE study when screening target drugs against cancer cells. Orders can be created without having to specify labware so that researchers can save time and ease protocol library management. Additionally, Cellario controls all activities in the workflow, gathering both analytical and meta data and sending it to a LIMS for archiving and downstream analysis.
Conclusion
PAIRWISE shows promise in accurately predicting drug combinations that can be confirmed through in vitro tests and translate into in vivo and clinical settings to strengthen personalized medicine therapies and improve patient outcomes. To learn more about PAIRWISE, read the pre-print article at doi.org/10.1101/2024.11.04.621884.
Fully integrating hardware and software in a HighRes automated system, including use of a pre-configured application ready work cell, creates a highly efficient and robust solution when confirming deep learning drug combination predictions in an in vitro setting. Cellario control boosts hands-free efficiencies in lab operations while enhancing seamless communication between hardware, software, and researchers. Contact your local HighRes expert to learn how our hardware and software can benefit your research workflows.